Hello and welcome to this video. In this video, we will be discussing how to run a log-log regression and interpret the regression coefficient estimate results. Let's begin by considering a model where the independent variables (education, experience, and age) and the response variable (wage) are all in log terms. Suppose we have collected some data and ed several regressions, resulting in the following regression results: - For the intercept (beta naught), the estimate is 1.5. - For the education coefficient (beta 1), the estimate is 3.3. - For the experience coefficient (beta 2), the estimate is 1.2. - For the age coefficient (beta 3), the estimate is 0.4. To interpret these results, we need to make a few assumptions. Firstly, the assumptions of the log-log regression model need to hold. Secondly, the regression coefficient estimates need to be statistically significant and practically significant, meaning they have a noticeable effect. Lastly, when interpreting the effect of education (beta 1) on wages, we need to hold the other variables (experience and age) constant in a multivariable regression. Now let's focus on interpreting the beta 1 coefficient. We can start by taking the partial derivative of the model with respect to education. Since we have log wages, the derivative of log X is DX divided by X. So, the derivative of log wages is the change in wage divided by wages. Similarly, the derivative of log education multiplied by beta 1 gives us the change in education divided by education times beta 1. One important thing to note is that the change in wage divided by wage (D wage / wage) represents the percent change in wages. Thus, we can multiply the equation by 100 to get the percent change in wages on one side and the percent change in education on the other side. Reinterpreting the equation, we can...
Award-winning PDF software
Video instructions and help with filling out and completing Are Form 8865 Functional
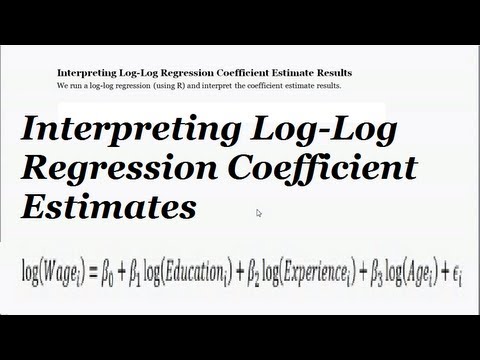